Five Ways Machine Learning is Revolutionizing Silicon Ingot Manufacturing and Beyond
Enhancing efficiency, supporting technicians, and minimizing errors, the future of chip manufacturing is here
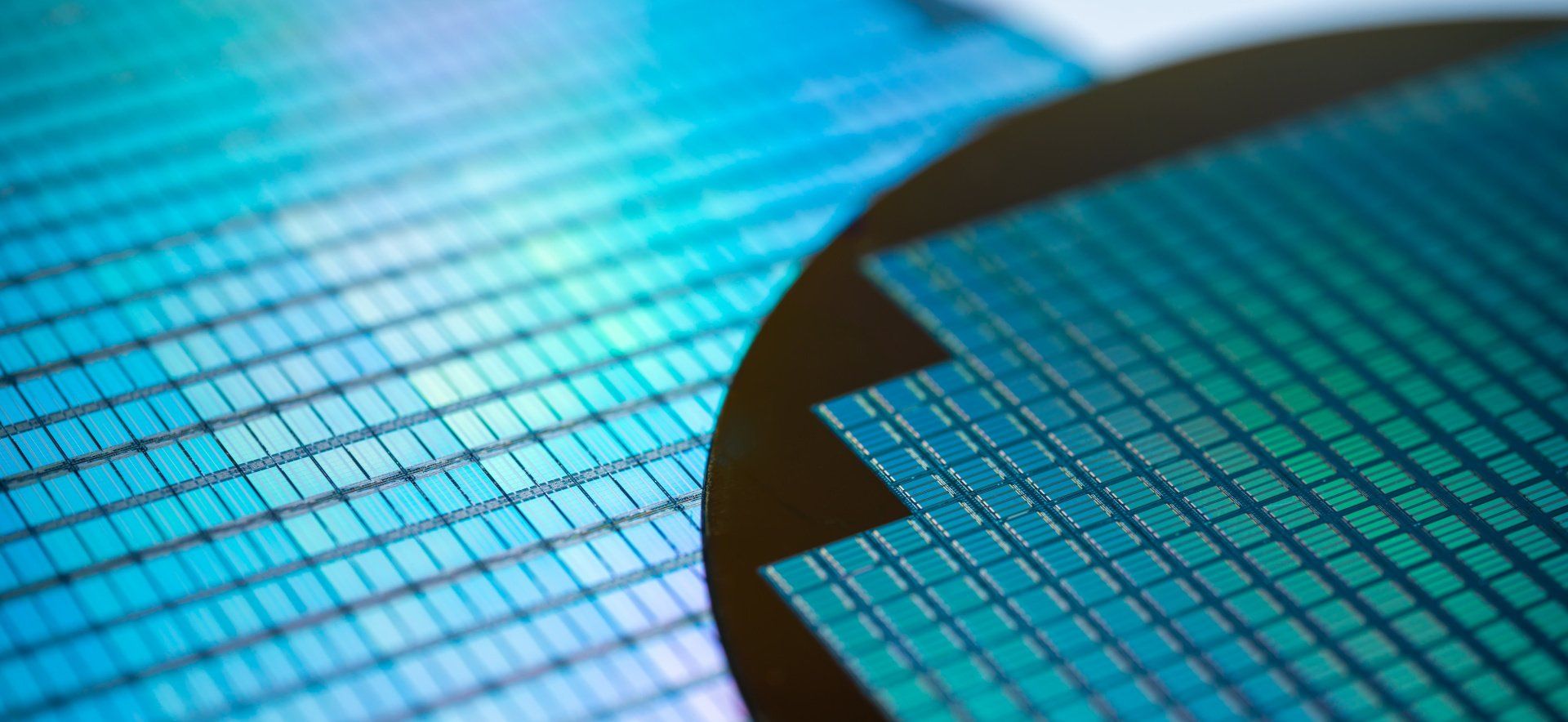
Silicon chip manufacturing, starting with silicon ingot manufacturing, has long been a complex and intricate process. As technology continues to evolve and the demand for smaller, more powerful devices increases, the pressure is on for chip manufacturers to keep up. Enter machine learning. This powerful technology is poised to transform the landscape of silicon ingot manufacturing, chip production, and everything in between, offering innovative solutions that improve efficiency, support technicians, and minimize errors. Here are five ways machine learning is revolutionizing the industry:
- Predictive Maintenance: Ensuring Uptime and Efficiency in Ingot and Chip Production
Machine learning algorithms can monitor and analyze equipment data in real-time, providing valuable insights into the health and performance of manufacturing tools. This is particularly relevant during the silicon ingot manufacturing stage, where equipment such as crystal pullers are used to produce the ingot neck and other critical parts of the silicon ingot. By enabling manufacturers to predict and prevent equipment failures, machine learning reduces downtime and ensures a more efficient production line. Optimizing maintenance schedules and addressing issues before they escalate helps manufacturers save time and money while maintaining high levels of productivity. - Process Optimization: Maximizing Yield and Quality
The manufacturing process for silicon ingots and chips involves numerous steps, with each stage having the potential to introduce defects. Machine learning can analyze vast amounts of data generated during production to identify patterns and correlations that human operators might miss. By pinpointing inefficiencies and determining optimal process parameters, machine learning can help manufacturers fine-tune their operations, resulting in higher yields, reduced waste, and better quality ingots and chips. - Anomaly Detection: Minimizing Errors and Rework
Machine learning models can be trained to recognize anomalies in data sets, including those related to silicon ingot manufacturing and the ingot neck formation. By continuously scanning production data, these models can identify and flag potential issues early, allowing technicians to address problems before they become costly mistakes. This reduces the need for rework and ensures that ingots and chips meet quality standards, all while minimizing production delays and maintaining customer satisfaction. - Enhanced Decision Support for Technicians
Machine learning can provide technicians with powerful decision support tools, helping them make more informed decisions based on real-time data. By leveraging machine learning algorithms to analyze complex data sets, technicians can gain insights into equipment performance, process parameters, and potential defects in both silicon ingot manufacturing and chip production. This valuable information allows them to take corrective actions proactively, ensuring that the manufacturing process remains on track and that quality remains high. - Automated Quality Control: Streamlining Inspection and Testing
Quality control is a crucial aspect of silicon ingot manufacturing and chip production, and machine learning is playing a pivotal role in automating this process. By implementing machine learning-based inspection systems, manufacturers can quickly and accurately detect defects in ingots and chips, reducing the need for manual inspection and speeding up the quality control process. These systems can also learn from their mistakes, continuously improving their accuracy and making the quality control process more efficient over time.
Machine learning is changing the game for silicon ingot manufacturing and chip production, offering transformative solutions that improve efficiency, support technicians, and minimize errors. By harnessing the power of machine learning, manufacturers can optimize processes, enhance decision-making, and streamline quality control, ensuring that they remain competitive in an increasingly demanding market. As technology continues to advance, we can expect even more innovative applications of machine learning in the silicon ingot manufacturing and chip production industries, driving productivity and performance to new heights.
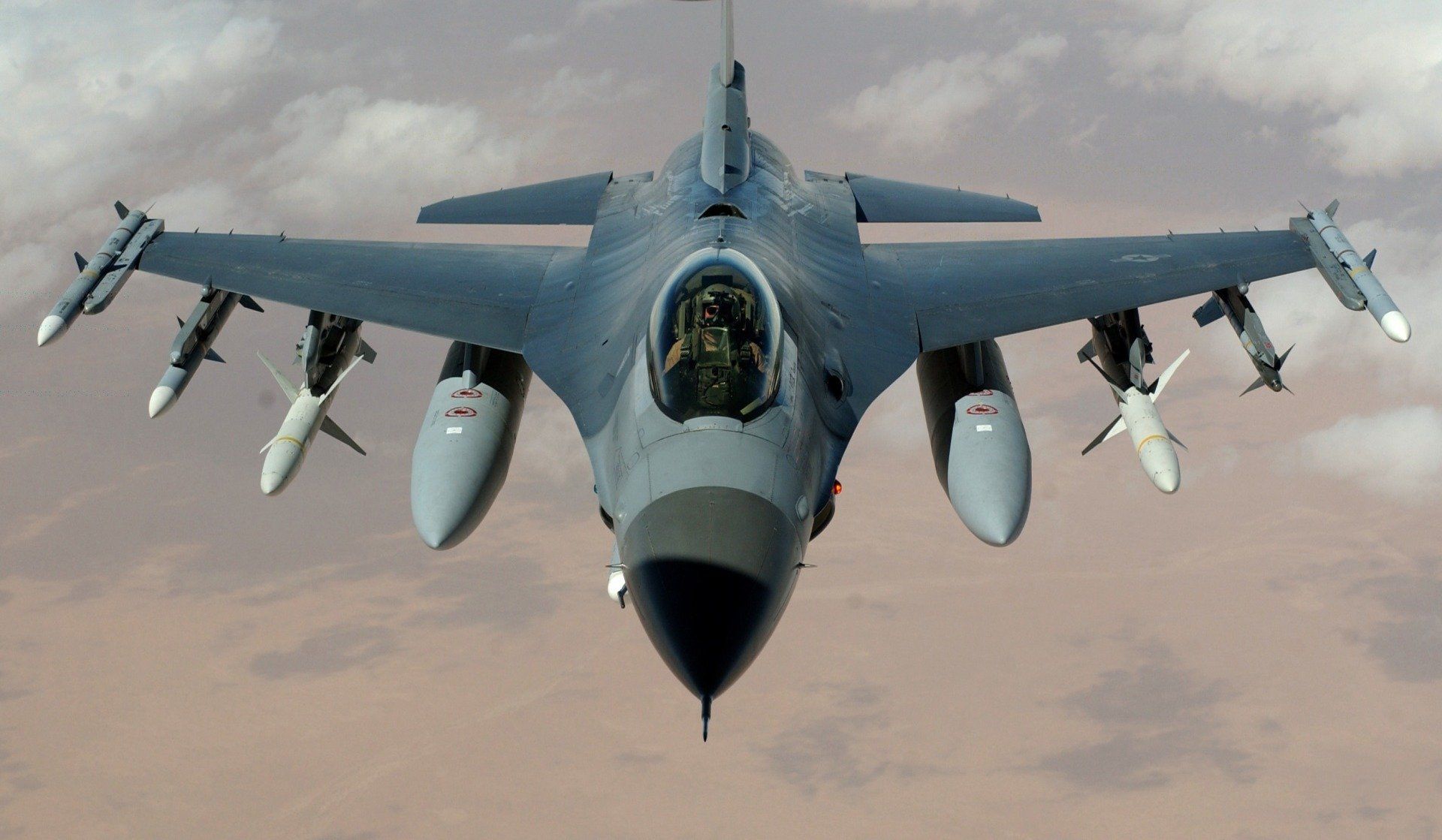
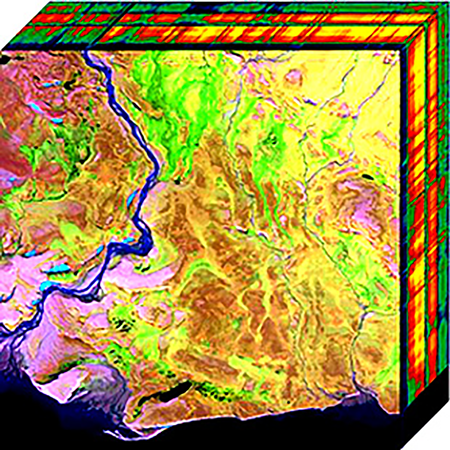