What are Spiking Neural Networks (SNNs)?
Spiking Neural Networks in ISR: Advantages and Applications
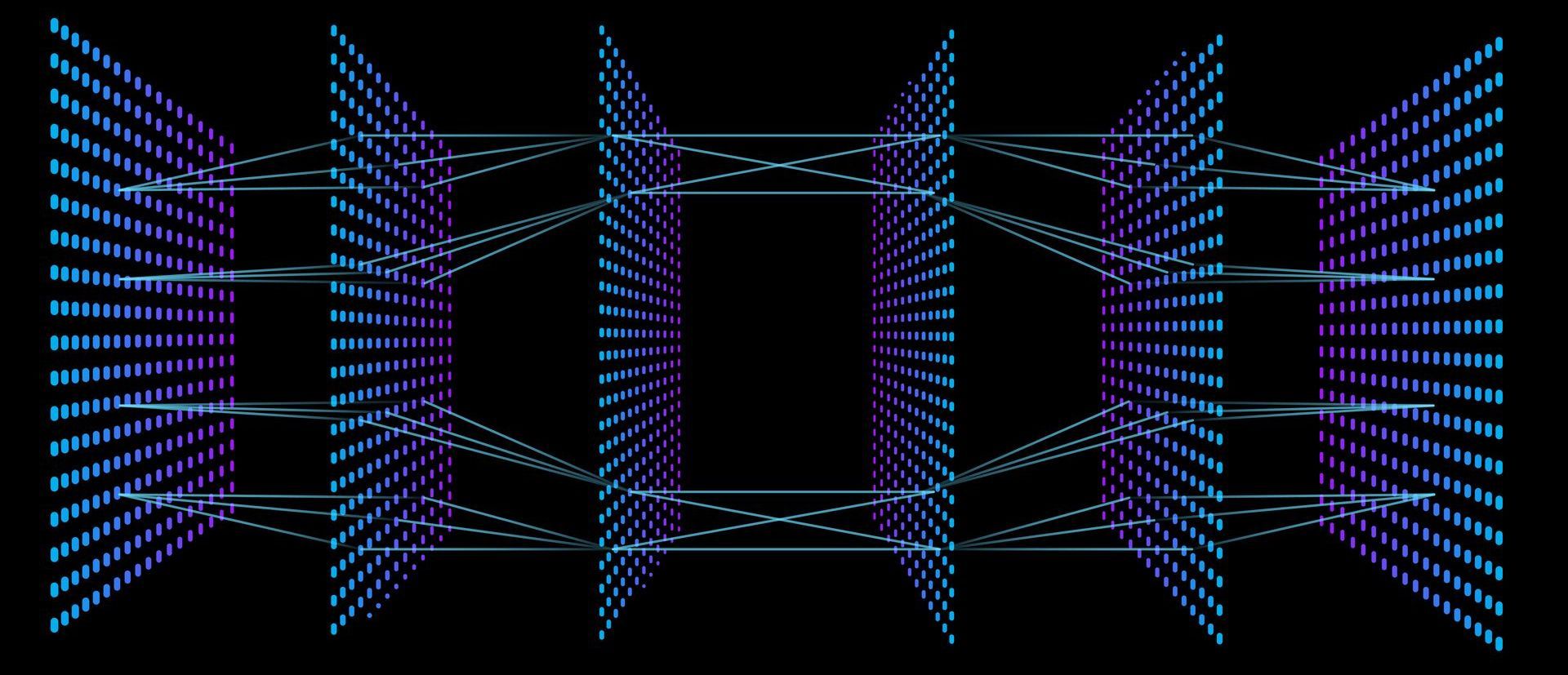
Why SNNs are a Game Changer for Real-Time Processing of Noisy Data
Machine learning algorithms are becoming increasingly popular in various fields, including intelligence, surveillance, and reconnaissance (ISR). Among these algorithms, spiking neural networks (SNNs) have unique properties that make them particularly well-suited for certain applications.
Let's take a closer look at what sets SNNs apart from other types of machine learning algorithms.
- One of the key advantages of SNNs is their efficiency. SNNs are designed to be highly efficient in terms of memory and computation, which is essential for real-time applications that require speed and accuracy. Unlike other machine learning algorithms that require a separate training phase followed by a separate inference phase, SNNs can perform both tasks simultaneously, making them more efficient.
- Another advantage of SNNs is their robustness. SNNs can handle noisy, incomplete, or ambiguous data, which is common in ISR applications. For example, SNNs can process input from sensors that may have missing data or be affected by environmental factors such as weather or lighting conditions.
- But perhaps the most significant advantage of SNNs is their biological plausibility. SNNs are inspired by the structure and function of the brain, which makes them more biologically realistic than other types of machine learning algorithms. This is particularly important for applications that involve cognitive tasks, such as object recognition and decision-making.
Of course, SNNs are not without their limitations. They require specialized knowledge and expertise to develop and optimize, and they may not always be the best choice for every application. However, for certain applications, particularly those involving real-time processing of noisy data, SNNs offer unique benefits that cannot be found in other types of machine learning algorithms.
The field of machine learning is constantly evolving, and there are many different types of algorithms available to researchers and practitioners. While SNNs may not be the right choice for every application, they offer unique advantages that make them well-suited for certain tasks, particularly those in the field of ISR. With continued research and development, it's likely that SNNs will continue to play an increasingly important role in the world of machine learning and beyond.
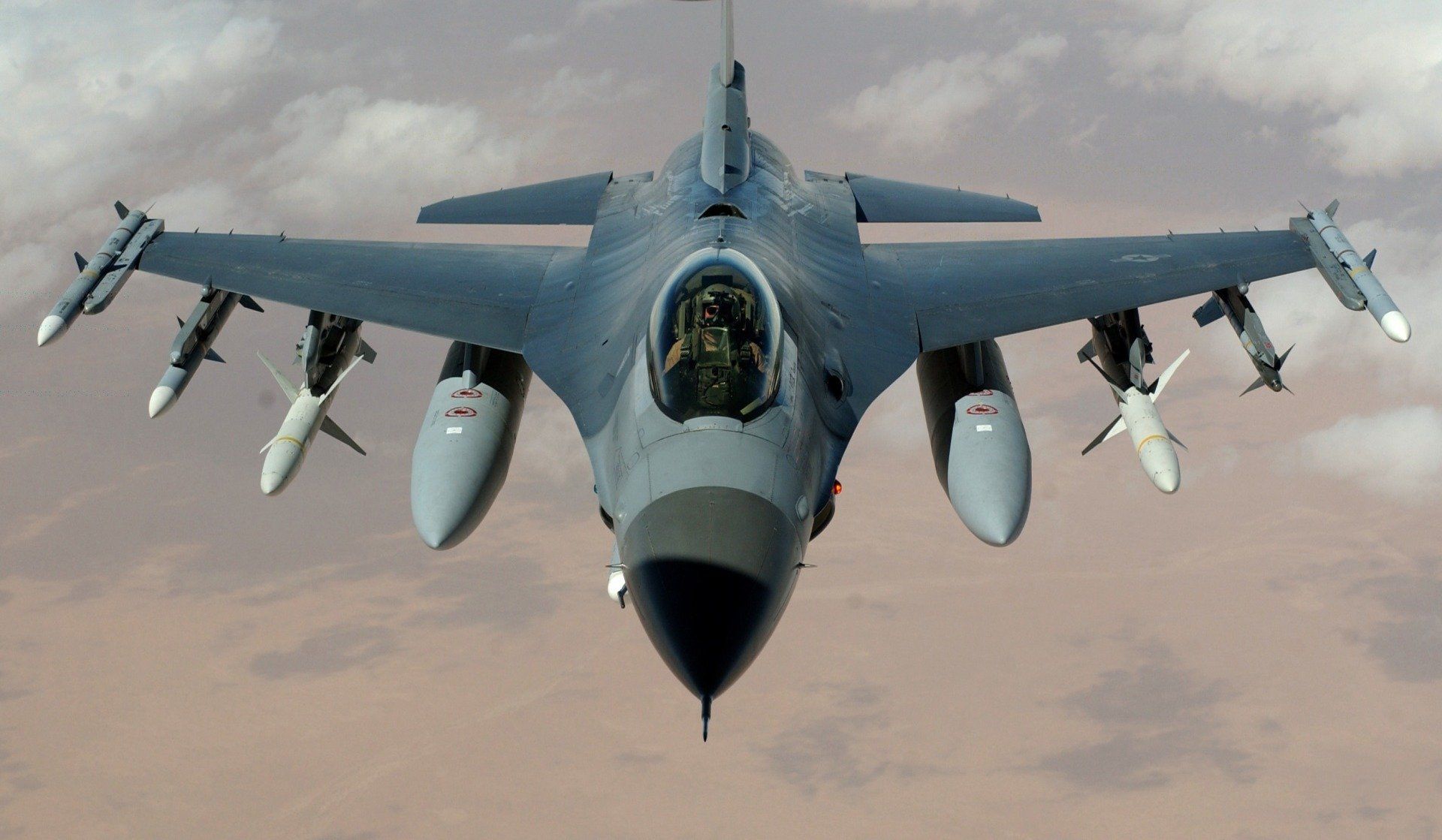
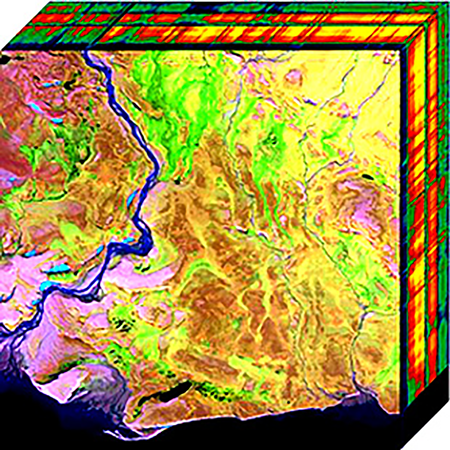