Five preprocessing strategies for enhancing Hyperspectral Data Analysis
Harnessing the Full Potential of Hyperspectral Imaging through Pre-Processing
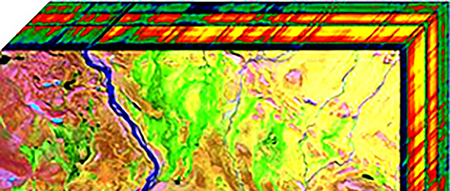
Hyperspectral imaging is a powerful tool in remote sensing, agriculture, environmental monitoring, and numerous other fields. However, the large volume and complexity of hyperspectral data can present challenges for researchers aiming to use machine learning effectively.
Pre-processing strategies can help you significantly improve your data quality, enabling more accurate and reliable machine learning applications.
For optimal results from hyperspectral data analysis, it is crucial to clean and prepare the data before using it in machine learning algorithms. Concentrate on noise reduction, spectral calibration, spatial registration, atmospheric correction, and dimensionality reduction, to optimize your data and take full advantage machine learning’s capabilities.
Here are five steps to help you get started:
- Noise reduction: Utilize techniques such as principal component analysis (PCA), wavelet denoising, or total variation denoising to minimize sensor noise and preserve vital spectral information.
- Spectral calibration: Perform calibration using known reference materials or established methods like the empirical line method or flat field correction to ensure consistent and accurate spectral data.
- Spatial registration: Correct misalignments and geometric distortions by aligning hyperspectral data with a reference image or coordinate system using ground control points or image-to-image registration techniques.
- Atmospheric correction: Account for atmospheric effects, such as scattering and absorption, to retrieve surface reflectance values using methods like the Fast Line-of-sight Atmospheric Analysis of Spectral Hypercubes (FLAASH) or the Atmospheric and Topographic Correction (ATCOR) algorithm.
- Dimensionality reduction: Retain the most informative spectral bands while reducing data size and computational requirements using techniques like PCA, minimum noise fraction (MNF), or independent component analysis (ICA). Outside of feature selection methods, be sure to use expert domain knowledge to aid in retaining the bands of most importance.
These five pre-processing strategies can help you substantially enhance the quality of your hyperspectral data, allowing for more accurate and reliable machine learning applications. Properly cleaned and prepared data helps you overcome the "curse of dimensionality" and ensures that the information fed into machine learning algorithms is consistent, accurate, and free from noise. As a result, you can fully harness the potential of hyperspectral imaging in your domain, drive innovation and facilitate better decision-making.
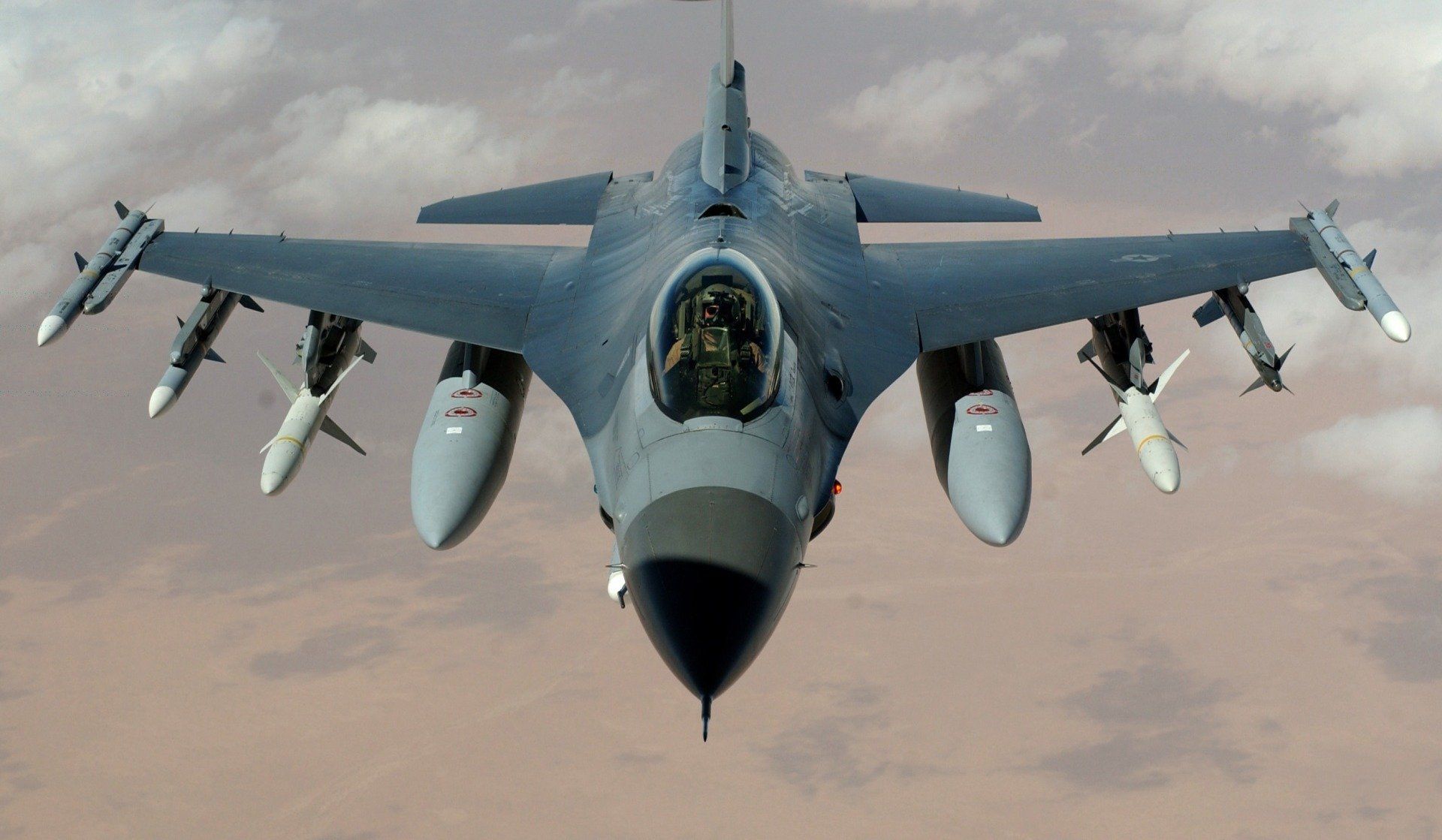
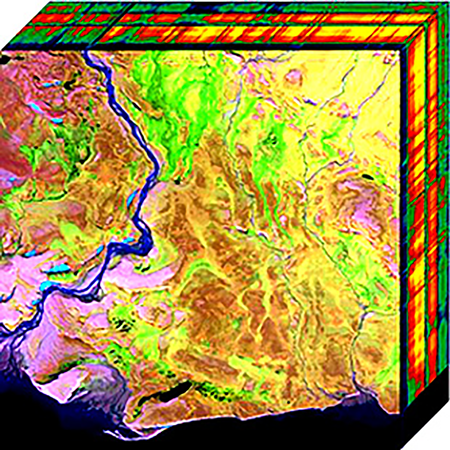